The world of private equity has long been perceived as a black box, its returns a puzzle for traditional financial analysts. But a cutting-edge study by Tausch and Pietz, featured in the Journal of Finance and Data Science, uses the power of machine learning to illuminate this intricate landscape.
Machine Learning Private Equity Returns
C. Tausch, M. Pietz
Journal of Finance and Data Science (Volume 10, December 2024, 100141)
Challenging the “Factor Zoo” with Model Combination
Pinpointing the factors that drive returns is an ongoing debate even in public markets, leading to what’s been dubbed the “factor model zoo.” This uncertainty is magnified in private equity. To address this challenge, the authors employ a novel approach: model combination. Inspired by ensemble learning, they combine multiple two-factor models, creating a robust public factor model that accounts for the ambiguity in factor selection. Each model is estimated using various parameters and data subsets. They consider key factors like market risk premium, size, value, dividend yield, and quality.
Beyond Beta: Decoding Idiosyncratic Returns
Traditional private equity analysis often hinges on Net Asset Value (NAV) data, which can be misleading due to infrequent valuations and potential smoothing of returns. Tausch and Pietz avoid this issue by directly analyzing cash flows, using a technique based on the work of Driessen et al. They then use componentwise L2 boosting to estimate the error terms – the portion of returns not explained by public factors. This method focuses on residual errors, iteratively refining the model to uncover the unique, often hidden, drivers of private equity performance.
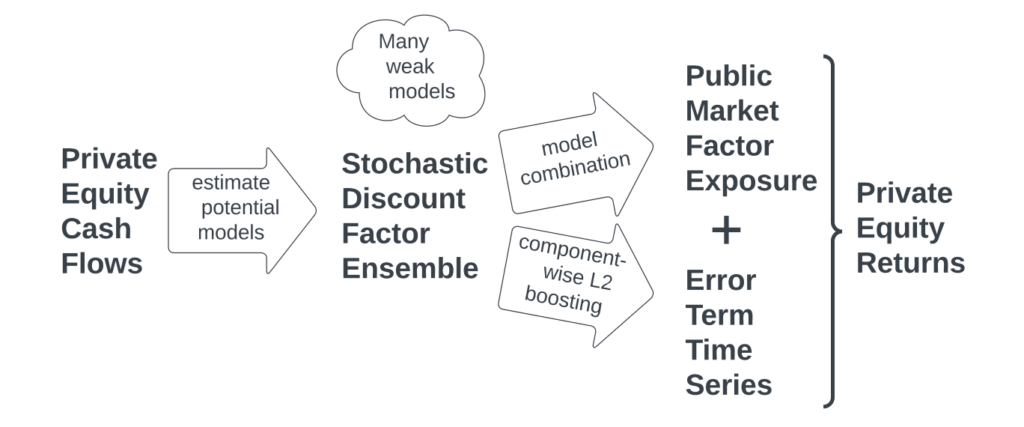
The Power of Sparsity: Illuminating the Nature of Idiosyncratic Returns
A key finding is the sparsity of the error term series. This means many entries are zero, suggesting that idiosyncratic forces in private equity returns are event-driven or infrequent, not constantly present. This provides a more nuanced understanding of the dynamics at play.
Beyond Smoothed NAVs: A Cash Flow Perspective
The paper highlights the inherent limitations of using Net Asset Value (NAV) data to accurately depict private equity returns. NAV, by its nature, suffers from stale pricing. Private equity investments are not traded on public exchanges, so their valuations are updated infrequently, often quarterly. This means NAV doesn’t capture the immediate impact of market movements, creating a smoothed, delayed picture of performance.
This smoothing effect leads to autocorrelation in NAV return series. Essentially, one quarter’s return is heavily influenced by the previous quarter’s return, not solely by the underlying performance of the investments during that quarter. As illustrated in Figure 6, the autocorrelation for the first two lags in the NAV return series from providers like Cambridge Associates, Preqin, and Pitchbook is significantly higher than in the model-derived return series proposed in the paper.
To circumvent these limitations, Tausch and Pietz move away from NAV and instead directly analyze the cash flows of private equity funds. By focusing on the actual cash inflows (contributions) and outflows (distributions) of funds, they capture a more accurate and timely representation of performance.
The resulting total return time series, which combines the public factor model returns and the estimated error terms (Average 2-Factor Models + Errors), exhibits significantly lower autocorrelation than the NAV returns provided by leading data providers. This provides a clearer picture of the timing and magnitude of private equity returns, free from the distortions of stale pricing and smoothing.
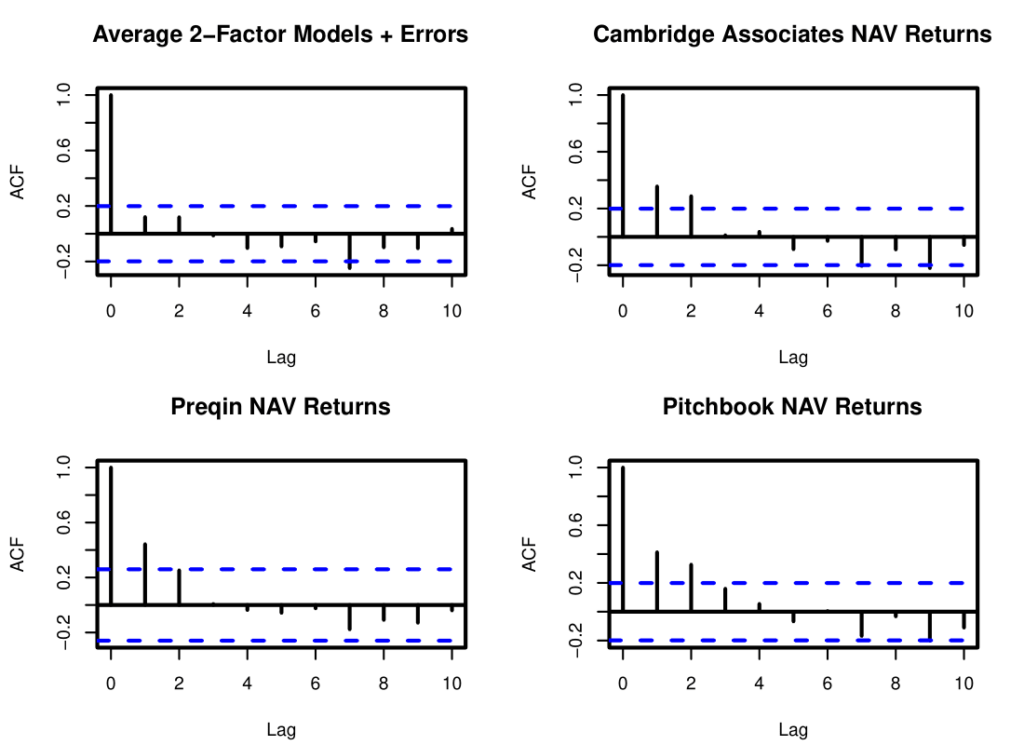
Unveiling the Buyout Fund Landscape
The authors apply their model to Buyout funds, with compelling results:
Accurate Return Replication: The combined model, incorporating public factors and idiosyncratic components, closely mirrors actual NAV returns reported by industry leaders like Cambridge Associates, Preqin, and Pitchbook.The model achieves a 79% Pearson correlation with the Cambridge Associates NAV return series.
Addressing Smoothing and Staleness: Model-derived returns show significantly lower autocorrelations than traditional NAV returns, resolving the issues of smoothing and staleness inherent in NAV-based analysis. This provides a clearer and more accurate picture of Buyout fund returns.
Shifting Dynamics: Before 2010, idiosyncratic returns were dominant in Buyout fund performance; post-2010, public factors took center stage. The average annualized idiosyncratic return for Buyout funds was 7.7% before 2010, plummeting to -0.4% after 2010.
Cumulative returns for BO funds
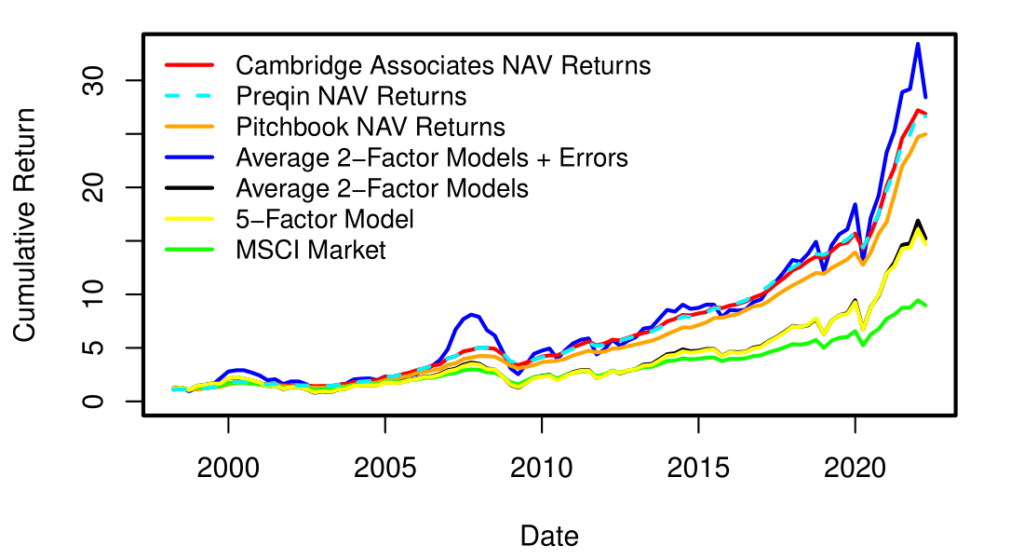
Promising: The model’s predicted returns (blue line) come fairly close to the NAV returns (red, cyan, orange).
Predicted idiosyncratic returns for BO funds
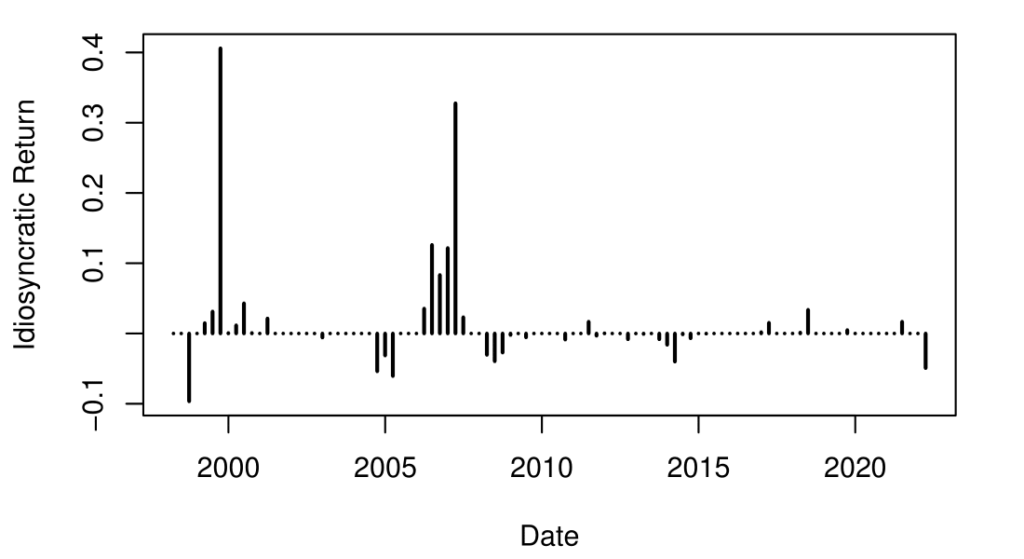
Interesting: The sparse idiosyncratic returns seem to be especially important in the Dot-Com Bubble 2000 and the Great Financial Crisis of 2007 to explain BO fund returns.
Rethinking “Alpha” and Empowering Investors
This research has profound implications for investors, fund managers, and academics:
Transforming Benchmarking and Risk Management: The model provides a standardized and robust framework for benchmarking private equity performance against public markets, facilitating more informed investment decisions and risk management strategies.
Challenging “Alpha”: By separating systematic and idiosyncratic returns, the model challenges the traditional notion of “alpha” in private equity. It suggests that much of the perceived outperformance may stem from specific risk exposures and market conditions rather than superior manager skill.
Unlocking New Avenues of Inquiry: The use of machine learning paves the way for deeper exploration of private equity returns, potentially leading to more sophisticated investment strategies.
Transparency and Future Refinements: Addressing Model Limitations
The authors acknowledge limitations, particularly the potential for extreme error term estimates at the beginning and end of time series. They recommend trimming these outliers or employing return caps. Additionally, they suggest exploring more advanced machine learning techniques to further refine the model.
Curious? Doubts? Convinced?
Read and download the full paper for free: Tausch and Pietz - Machine Learning Private Equity Returns in the Journal of Finance and Data Science (Volume 10, December 2024, 100141).
We highly value your feedback! To get in touch, feel free to comment!